티스토리 뷰
이전 포스트에서도 계속 언급했다시피, 딥러닝 모델을 모바일이나 임베디드 환경에서 그대로 돌리기 어려운 이유는 일반 PC와 달리 메모리나 성능, 저장공간 등의 제한이 있기 때문이다. 이 때문에 해당 모델을 어떻게 최적화(Optimization)하느냐가 임베디드 환경상에서도 최적의 성능을 낼지 여부를 결정하는 요소가 된다. Tensorflow Lite도 결국은 Model deploy시 이 최적화를 해주는 기능이 포함되어 있는 것인데, 이 때 Quantization이 적용된다.치ㅑ
Quantization은 간단히 말해서 Neural Network의 내부 구성이나 표현되는 형식을 줄이는 과정을 말한다. 예를 들어서 현재 구성된 Neural Network의 weight이나 activation output이 32bit floating point로 표현되는 형식이라고 치자. 참고로 만약 IEEE 754 형식의 32 bit floating point를 표현형식으로 쓴다면 구성은 다음과 같다.
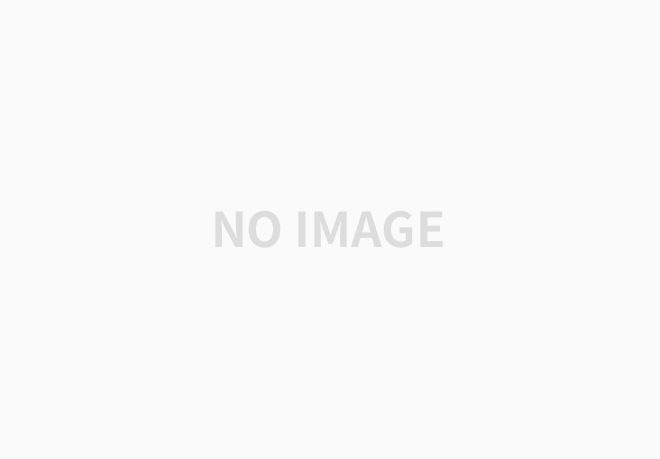
물론 weight 하나하나의 precision이 최종 output에 영향을 많이 끼치겠지만, 임베디드 환경에서는 앞에서 언급한 바와 같이 제한된 저장공간으로 인해서 이를 다 활용하기가 어렵다. 대신에 위의 표현식을 8bit integer 형으로만 표현한다면, 물론 기존의 모델보다는 성능이 안나와도 적어도 표현 형식이 32bit -> 8 bit으로 바꾸면서 모델의 크기를 크게 줄일 수 있을 것이다.
Tensorflow Lite에서 지원하는 Quantization은 Quantization-aware training이라는 Training 중에 수행되는 Quantization과 Post-Training Quantization이라 하여 Training 후에 Quantization을 수행하는 방식 두가지가 있는데, 이중 Post-Training Quantization에 대해서 조금더 살펴보겠다.
Post-Training Quantization은 방금전에 설명한 그대로 Training을 완료한 후에 CPU나 Edge TPU/GPU같은 Hardware Accelerator의 latency를 개선시키면서 accuracy에 대한 손해를 최소화한 기법이다. 공식 페이지에 설명된 대로라면 일반 tensorflow에서 학습한 model을 TFLiteConverter를 통해서 tflite model로 변환시킬때 이 작업이 이뤄진다. Quantization Option으로는 다음과 같이 제공된다.
TF Lite Option | Technique Used | Benefits | Applied Hardware |
OPTIMIZE_FOR_SIZE | Weight Quantization ("Hybrid operation") |
4x model smaller, 2-3x speedup, Accuracy |
CPU |
DEFAULT | Integer Quantization | 4x model smaller, More speedup |
CPU, Edge TPU, etc |
DEFAULT + tf.lite.constants.FLOAT16 |
Float16 Quantization | 2x model smaller, Potential GPU Acceleration |
CPU/GPU |
("Hybrid operation"이란 Float Quantization과 Integer Quantization이 결합된 형태로 operation 별로 floating-point 연산과 Integer 연산을 할수 있도록 최적화를 거친 것을 말한다.)
딱 보면 Hybrid operation이 좋아보이지만, Edge TPU와 같은 Hardware Accelerator는 Floating point operation이 지원하지 않기 때문에 Integer quantization같은 다른 option을 선택해야 한다. 그리고 이 모든 Quantization process는 TFLiteConverter에서 이뤄진다.
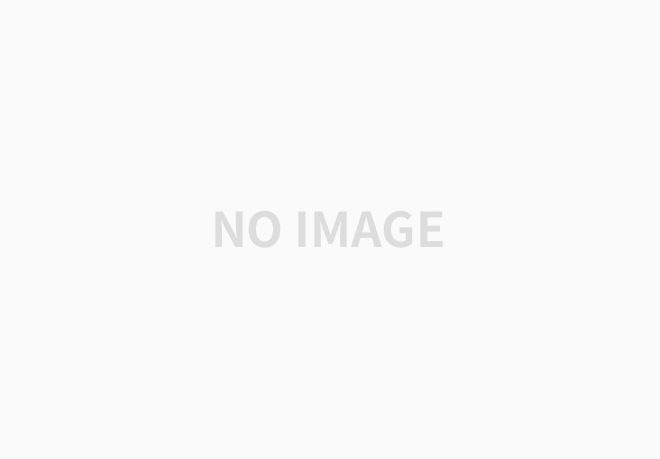
Hybrid Operation에 대한 예제는 다음과 같다.
import tensorflow as tf
import pathlib
x = [-1, 0, 1, 2, 3, 4]
y = [-3, -1, 1, 3, 5, 7]
# Create a simple Keras Model
model = tf.keras.models.Sequential([
tf.keras.layers.Dense(units=1, input_shape=[1])
])
model.compile(optimizer='sgd', loss='mean_squared_error')
model.fit(x, y, epochs=500)
# Save model to export_dir
export_dir = '.'
tf.saved_model.save(model, export_dir)
# Convert the model to tflite model format
converter = tf.lite.TFLiteConverter.from_saved_model(export_dir)
converter.optimizations = [tf.lite.Optimize.OPTIMIZE_FOR_SIZE]
tflite_model = converter.convert()
# Save the model
tflite_model_file = pathlib.Path('./a.tflite')
tflite_model_file.write_bytes(tflite_model)
위의 예제는 맨 처음에 다뤘던 예제와 동일하게 single node로 구성된 1 Layer ANN을 500회만큼 학습시키고, 이를 기본 제공하는 model 형식으로 저장했다.(물론 이때는 'saved_model.pb'라는 파일이 생성된다.) 그후에 앞에서 소개한 OPTIMIZE_FOR_SIZE라는 optimization option을 정의한 후 TFLiteConverter를 통해 변환시키고 이에 대한 출력 model을 'a.tflite'라는 파일로 저장했다. 결과는 최종 출력 model의 크기를 비교해보면 사이즈가 약 1/4 정도 줄어든 것을 확인할 수 있다.
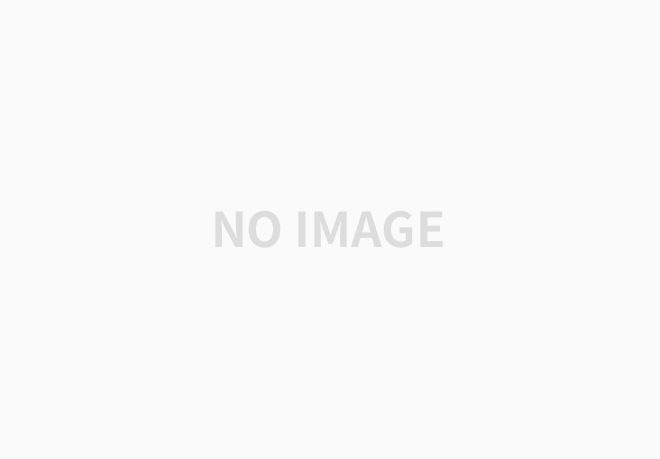
(Optimization Mode를 바꾼 예제도 제공되나 수정이 필요해서 추후 내용 기재 예정)
최종적으로 Post Training Quantization을 수행할 때, 어떤 option을 선택해야 할지에 대해서 설명을 해준 Decision Tree가 있다.
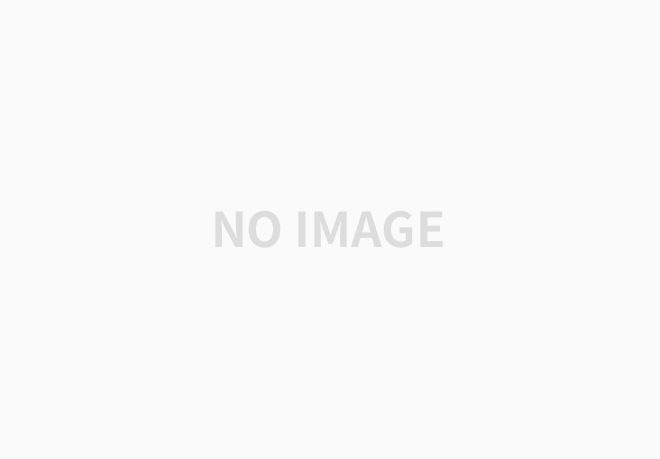
우선 처음 다뤄야 할 내용은 과연 내가 만든 model에 Optimization이 필요한가 여부이다. 단순히 high performance를 제공하는 embedded device에 올리는 정도라면 그냥 Converter의 default option을 활용해서 변형해주는 것만으로도 충분하다. 하지만 이외에 GPU나 TPU와 같은 Hardware Accelerator를 써야 하거나 아예 Hardware Limitation이 있는 경우는 앞에서 소개한 Post-Training Quantization이 필요하다. 특히 GPU같은 경우는 보통 16 bit floating point 연산에 맞춰져 있기 때문에 앞에서 예제로 소개한 것과 같이 float16 quantization을 통해서 optimization이 수행되어야 한다. 반대로 GPU가 아닌, integer 연산만 지원하는 MCU나 TPU같은 경우는 Integer Quantization이 이뤄져야 하는데, 여기서 RepresentativeDataset이라는 것이 있는지 여부를 체크해야 되는 경우가 있다. 이건 말 그대로 optimization이 이뤄진후, 즉 TFLiteConverter에 의해서 converting된 model이 optimization이 잘됬는지 여부를 확인할 수 있게끔 만들어진 dataset이다. 예제를 살펴보면 보통 Tensorflow Dataset (tfds)를 일종의 generator형식으로 만든 형태로 들어가게 된다.
물론 위에서 소개한 Post-Training Quantization이 잘 동작하기도 하지만, 몇몇 케이스에 대해서는 또다른 Quantization 방법인 Quantization-Aware Training이 좋은 성능을 나타내는 경우도 있기 때문에, Post-Training Quantization에 대한 결과가 안 좋은 경우는 후자의 방법을 취해보는 것도 좋을거 같다.(그런데 이 방법은 공식 문서에도 잘 소개되어 있지 않다. github 참고)
'Study > EmbeddedSystem' 카테고리의 다른 글
[Embedded][DL] TF Microcontroller Challenge (0) | 2021.06.19 |
---|---|
[Embedded][DL] Tensorflow Lite - Example (0) | 2019.11.15 |
[Embedded][DL] Tensorflow Lite - Introduction (0) | 2019.11.15 |
[Embedded] Performance Measures (1) (0) | 2017.07.10 |
[Embedded] Serial Communication (0) | 2017.07.10 |
[GPIO] GPIO Port Mode(Direction) Register (0) | 2017.06.20 |
[MOOC] Obstacle Avoidance Robot in CyberSim (0) | 2014.05.25 |
- Total
- Today
- Yesterday
- RL
- End-To-End
- SketchFlow
- windows 8
- ColorStream
- Pipeline
- Distribution
- 딥러닝
- Kinect
- Offline RL
- Kinect for windows
- processing
- 한빛미디어
- Gan
- Off-policy
- DepthStream
- Policy Gradient
- 강화학습
- arduino
- Windows Phone 7
- PowerPoint
- dynamic programming
- Kinect SDK
- reward
- Variance
- 파이썬
- 인공지능
- TensorFlow Lite
- bias
- Expression Blend 4
일 | 월 | 화 | 수 | 목 | 금 | 토 |
---|---|---|---|---|---|---|
1 | 2 | 3 | 4 | 5 | ||
6 | 7 | 8 | 9 | 10 | 11 | 12 |
13 | 14 | 15 | 16 | 17 | 18 | 19 |
20 | 21 | 22 | 23 | 24 | 25 | 26 |
27 | 28 | 29 | 30 |