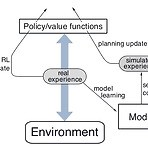
(해당 포스트는 Coursera의 Sample-based Learning Methods의 강의 요약본입니다) 이전 포스트를 통해서 강화학습 상에서의 Model의 정의에 대해서 소개하고, Model을 통해서 생성한 simulated experience를 바탕으로 model을 update하는 Planning에 대해서 다뤘다. 사실 이런 planning과정과 별개로 실제 environment로부터 얻은 experience를 바탕으로 update하는 것을 Direct RL이라고 표현한다. 이번 포스트에서 소개할 Dyna algorithm (sutton)은 앞에서 소개된 Planning과 Direct RL이 결합된 형태로 되어 있다. 우선 기존의 Q-learning과 마찬가지로 실제 environment로부터 e..
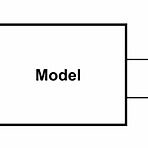
(해당 포스트는 Coursera의 Sample-based Learning Methods의 강의 요약본입니다) 강화학습을 공부하다보면 state나 action같은 기본 notation만큼이나 자주 나오는 단어가 Model이 아닐까 생각된다. 이전 포스트에서 Monte Carlo method나 Temporal Difference Learning을 다뤘고, 이 둘의 차이가 여러가지가 있지만, 그래도 넓은 관점에서 보자면 두 알고리즘은 Model이 있냐(Model-based) 없냐(Model-free)로 나눠서 볼 수 있다. sutton 책에 있는 표현을 가져오자면 Model-based RL은 planning에 초점이 맞춰져 있고, Model-free RL은 learning에 중점을 두고 있다. 그럼 여기서 말..
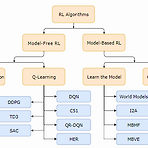
(본 글은 OpenAI Spinning Up을 개인적으로 정리한 글입니다. 원본) Part 2: Kinds of RL Algorithms — Spinning Up documentation We’ll start this section with a disclaimer: it’s really quite hard to draw an accurate, all-encompassing taxonomy of algorithms in the modern RL space, because the modularity of algorithms is not well-represented by a tree structure. Also, to make somethin spinningup.openai.com RL Algorithm의 ..
- Total
- Today
- Yesterday
- DepthStream
- Gan
- Windows Phone 7
- Pipeline
- Off-policy
- TensorFlow Lite
- processing
- arduino
- Kinect
- RL
- Kinect for windows
- End-To-End
- Policy Gradient
- bias
- SketchFlow
- Offline RL
- Expression Blend 4
- dynamic programming
- 한빛미디어
- 강화학습
- Kinect SDK
- 파이썬
- reward
- Distribution
- 딥러닝
- PowerPoint
- ColorStream
- windows 8
- Variance
- 인공지능
일 | 월 | 화 | 수 | 목 | 금 | 토 |
---|---|---|---|---|---|---|
1 | 2 | 3 | 4 | 5 | ||
6 | 7 | 8 | 9 | 10 | 11 | 12 |
13 | 14 | 15 | 16 | 17 | 18 | 19 |
20 | 21 | 22 | 23 | 24 | 25 | 26 |
27 | 28 | 29 | 30 |