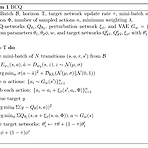
(논문의 의도를 가져오되, 개인적인 의견이 담길 수도 있습니다.) Off-Policy Deep Reinforcement Learning without Exploration - Fujimoto et al, ICML 2019 (논문, 코드) 요약 이 논문에서는 이미 모아져있는 고정된 dataset 상에서 강화학습 에이전트를 학습할 수 있는 알고리즘을 소개한다. 보통 강화학습은 exploration을 통해서 insight를 얻어내고, 이에 대한 경험으로 성능을 추출하는 형태로 되어 있지만, 고정된 dataset으로부터 학습하게 되면 exploration을 할 수 없기 때문에 성능을 얻어낼 요소가 부족하다. 이런 종류의 알고리즘을 Offline RL 혹은 Batch RL이라고 표현하고, 사실 이 알고리즘은 be..
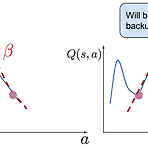
(해당 글은 U.C. Berkeley 박사과정에 재학중인 Daniel Seita가 작성한 포스트 내용을 원저자 동의하에 번역한 내용입니다) Offline (Batch) Reinforcement Learning: A Review of Literature and Applications Reinforcement learning is a promising technique for learning how to perform tasks through trial and error, with an appropriate balance of exploration and exploitation. Offline Reinforcement Learning, also known as Batch Reinforcement Learni..
- Total
- Today
- Yesterday
- Expression Blend 4
- TensorFlow Lite
- 한빛미디어
- 딥러닝
- Variance
- Kinect
- dynamic programming
- Distribution
- DepthStream
- Policy Gradient
- windows 8
- bias
- PowerPoint
- Offline RL
- Off-policy
- Windows Phone 7
- reward
- End-To-End
- Kinect SDK
- Kinect for windows
- ai
- arduino
- ColorStream
- RL
- SketchFlow
- processing
- Pipeline
- 파이썬
- Gan
- 강화학습
일 | 월 | 화 | 수 | 목 | 금 | 토 |
---|---|---|---|---|---|---|
1 | 2 | 3 | 4 | |||
5 | 6 | 7 | 8 | 9 | 10 | 11 |
12 | 13 | 14 | 15 | 16 | 17 | 18 |
19 | 20 | 21 | 22 | 23 | 24 | 25 |
26 | 27 | 28 | 29 | 30 | 31 |